ai and finance
AI-Powered Finance: New Practices, ROI and Future Outlook
This report explores the current state of AI in finance, including ROI for the business, the human impact of automation, what finance leaders should look to next, and actionable recommendations to help teams scale AI sustainably.
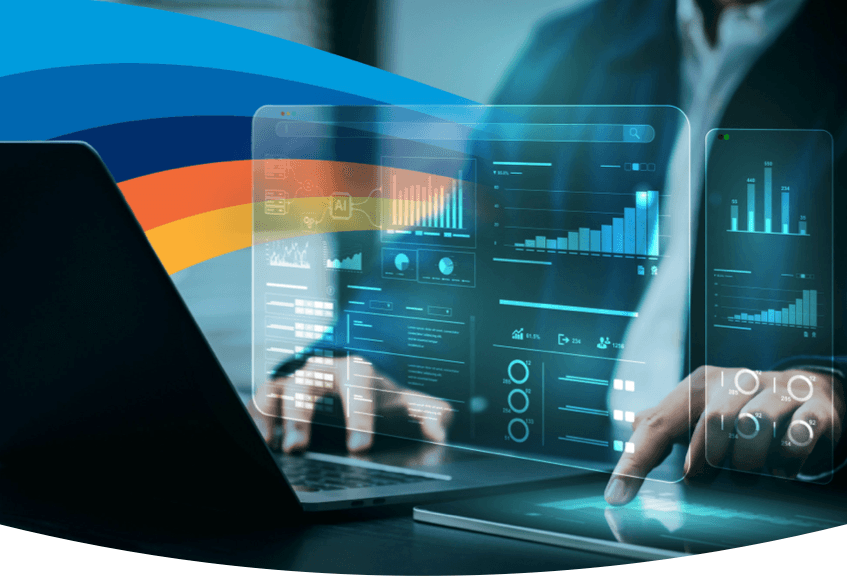
Executive summary
Finance teams are embracing AI, but the path to transformation is more nuanced than headlines suggest. Our latest survey of 1,500 finance professionals in the U.S. and UK reveals that organizations are actively investing in AI, and 66% have already made strategic purchases or hires. But adoption of AI within finance departments has significant room for growth, with just 35% of expense management processes being AI-driven.
The survey data shows that 74% of finance professionals say their company’s AI investments are delivering ROI that meets or exceeds expectations, and just 3% report outcomes that fall short. Yet despite these promising results, AI adoption is not yet a top priority for most finance leaders. Only 16% rank it as their department’s most pressing focus, lagging behind more traditional concerns like cost reduction (39%) and compliance or risk management (24%).
AI is also prompting teams to rethink how they measure success, driving broader changes across financial evaluation frameworks. Nearly three in four respondents report that tracking the ROI of AI has changed how they assess other areas of the business.
Still, there are hurdles. Concerns about data integrity, human oversight, and AI errors remain top of mind across all levels of the finance function. And while Gen Z and Millennial finance professionals are leading the charge in daily AI usage, only 39% of companies offer structured AI training.
AI is not a plug-and-play solution. But for finance teams willing to align technology with strategic thinking, AI offers a powerful lever that not only optimizes expense management but also enables it to evolve. From fraud detection and forecasting to accounts payable automation, early adopters of AI are already seeing time savings, stronger compliance, and enhanced operational efficiency.
This report explores the current state of AI in finance, including ROI for the business, the human impact of automation, and what finance leaders should look to next. It also includes actionable recommendations to help teams scale AI sustainably while aligning with Emburse’s mission for today’s leading companies: to simplify travel and expense (T&E) processes through intelligent automation.
Methodology
All figures presented in this report are from a comprehensive survey conducted by Talker Research, whose team members are accredited by the Market Research Society (MRS) and the European Society for Opinion and Marketing Research (ESOMAR). The research was fielded between March 24–31, 2025, using a random double-opt-in methodology to ensure data accuracy and respondent validity.
A total of 1,500 finance professionals participated in the study, including 1,000 respondents from the United States and 500 from the United Kingdom. All respondents were screened to confirm their active roles in financial functions within their organizations. This research provides a representative view of how U.S. and UK finance teams are investing in and measuring the ROI of AI across key operational areas.
Survey demographics
Respondents by age
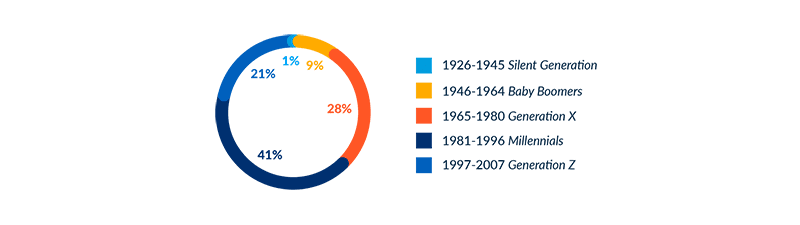
Respondents by seniority level
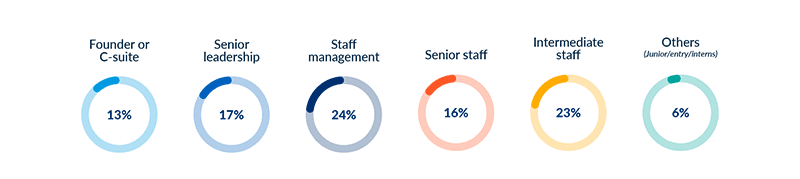
Respondents by organization size

The state of AI investment in finance
AI adoption in finance is real, but it’s still ramping up. While 66% of finance professionals report that their companies have made organizational investments in AI, adoption inside finance teams remains uneven.
On average, 18.4% of the finance budget is allocated to AI-related initiatives, signaling growing intent. The most common investments include buying enterprise software with AI features (54%), GenAI licenses for employees (48%), and AI-powered customer experiences (35%). Just over one in five companies (22%) have taken the bold step of building their own models or tools, a move that suggests deeper internal capabilities, investment, and ambition.
Top AI Investments
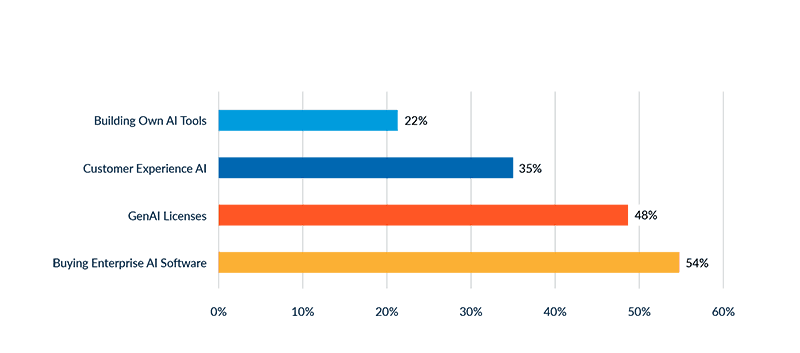
Despite these investments, AI adoption isn’t the biggest priority for most finance teams. Only 16% of finance professionals cited AI innovation as their department’s top focus, compared to 39% who prioritized cost-cutting and efficiency measures, and 24% focused on compliance and risk management. These figures suggest that while AI is on the roadmap, most teams are still treating it as an enabler, not a critical business process.
This perspective may be shifting, however. As the pace of innovation accelerates, so does the recognition that AI can elevate finance from a transactional function to a strategic partner. Adriana Carpenter, Chief Financial Officer at Emburse, shared that enabling teams to upskill and helping them truly understand the purpose of AI is key: “It’s about explaining the benefits to the finance function of being able to implement AI to improve their overall strategic position within the company and make better decisions.”
ROI expectations of AI
For most organizations, the return on AI investment meets expectations—and often exceeds them. According to the survey, 74% of finance professionals say the ROI of their company’s AI spend is as expected or better, while only 3% report outcomes that fell short.
Still, there’s no single formula for AI success. Nearly half (48%) describe their AI goals as realistic, while 24% describe them as ambitious. What’s even more telling is that 27% of companies are moving forward with AI initiatives despite concerns about ROI, reflecting a willingness to invest with a long-term view.
These long-term ambitions are reshaping how finance leaders evaluate performance across the organization. For example, 85% of respondents said measuring the ROI of AI has influenced how they assess other business areas. For some, this means adopting a longer-term strategic lens (30%). Others are shifting their focus toward operational efficiency (29%) or embracing real-time measurement methods (27%).
This evolution highlights the broader value of AI beyond dollars saved. AI is a force multiplier that enhances decision-making, improves risk controls, and speeds up processes —from reimbursements to audit trails. The return isn’t just financial—it’s operational, cultural, and strategic.
The finance professional’s experience
Across finance teams, day-to-day workflows are quietly evolving. On average, finance professionals now interact with AI 3.7 times a day, with 37% of their tasks involving some form of AI. For Gen Z and Millennial employees, that number jumps even higher, with over 40% of their work being AI-assisted, signaling a generational shift in how this technology is being integrated into the profession.
Despite its rapid adoption, the learning curve for AI remains unbalanced. While 62% of finance professionals feel confident in their team’s ability to use AI tools effectively, many organizations haven’t formalized its support: just 39% offer structured training, and 31% expect employees to self-learn. The result is a landscape where enthusiasm about AI often outpaces enablement.
Still, the sentiment is overwhelmingly positive. Nearly half (46%) of respondents say AI saves them time, and 27% feel it makes their role more strategic and less stressful. Only one in five believe AI won’t significantly change their role. And in sharp contrast to fears about automation, 85% reject the idea that AI will fully replace finance jobs.
However, trust and balance do matter. Almost one-quarter of finance professionals reported that they would leave their employer if AI risks were not taken seriously, and three in ten would consider leaving if their employer prioritized AI investment over human talent. These concerns point to a desire for responsible leadership, one that frames AI as a partner, not a replacement for human capabilities.
As finance professionals shift from data compilers to critical thinkers and strategic advisors, the role of AI becomes clearer: AI is not a shortcut, but rather a tool that elevates impact when implemented with intention and backed by thoughtful governance and training.
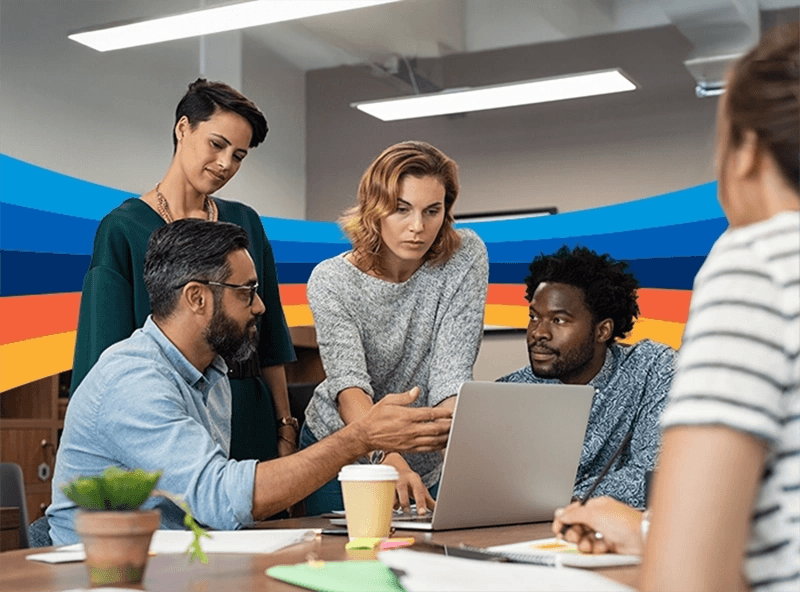
Expense management transformation
While the excitement and interest in AI continue to grow across finance functions, many organizations have yet to fully realize its value in expense management. Only 35% of respondents say their expense workflows are currently AI-driven, signaling significant opportunity for enhanced automation and spend optimization.
The most common application of AI in this area remains a minority, with only 40% of finance teams using AI for analyzing and reporting on spend data. Though this marks a positive shift toward data-driven decision-making, it also highlights a missed opportunity: few organizations are leveraging AI to automate complex, routine tasks across the full expense lifecycle. Fragmented systems persist as a core challenge, with many companies still managing T&E, vendor spend, and other expense categories across disconnected platforms. This siloed approach makes it difficult to gain a unified view of total spend, let alone to apply AI in an integrated and intelligent way to drive speed, accuracy, and compliance.
Despite these challenges, finance teams are already seeing strong returns in specific areas. When asked which AI-driven finance functions are delivering the highest ROI, forecasting and budgeting (26%), accounts payable and receivable automation (26%), and fraud detection (25%) emerged as the front-runners.
Top 3 AI-Driven Finance Functions Delivering the Highest ROI
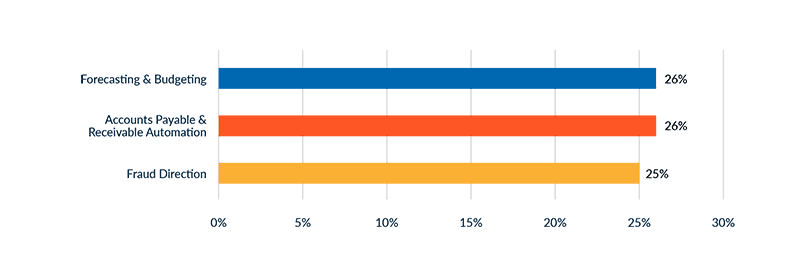
These results reinforce that AI is already driving measurable impact beyond reporting, but scaling that success requires a unified approach and integrated data infrastructure. As AI use cases mature and data ecosystems become more complex, the ability to adapt, scale, and integrate new capabilities over time will increasingly define the long-term success of AI in the finance function.
Key concerns and risk management
As finance leaders explore AI adoption, concerns around its implementation are shaping how teams approach integration. Nearly a quarter of respondents (24%) identified data security and privacy as their primary concern, underscoring the sensitive nature of financial data and the need for robust governance when implementing intelligent systems.
Skepticism around AI output
The survey results around trust in AI was another core theme: 18% pointed to the risk of AI making mistakes or introducing errors, while 10% expressed doubts about the reliability of AI-generated insights. These findings may reflect a broader anxiety about “black box” decision-making, where AI generates results that can’t be easily explained, audited, or corrected.
Concerns around workforce impact were also prominent, with 18% of respondents worried about how AI adoption could affect their roles. While AI promises productivity gains, the perception of automation as a threat remains a barrier to widespread acceptance.
Investment uncertainty
Finally, 9% of finance professionals cited implementation costs as a top concern, suggesting that finance leaders remain cautious about the investment required to adopt and scale AI across the finance function.
Taken together, these results show that while interest in AI is growing, finance teams remain mindful of the risks that come with adoption. As organizations move forward, a proactive strategy addressing these concerns will be key to building trust, ensuring transparency, and unlocking AI’s full potential in a responsible, secure, and sustainable way.
Biggest Concerns Regarding AI in Finance
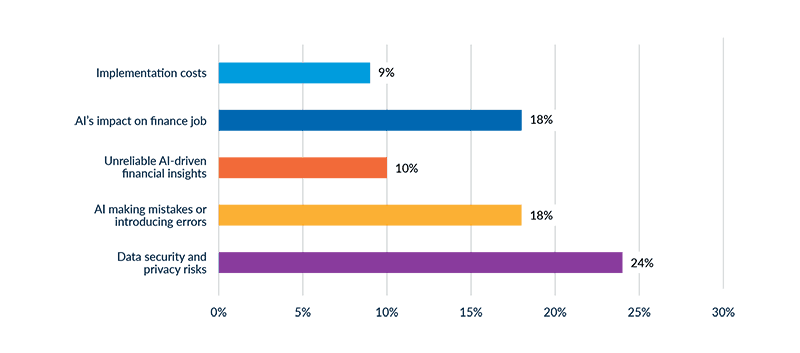
Future outlook and strategic considerations
As AI capabilities mature, finance leaders expect its impact to extend beyond current use cases. Financial planning and forecasting is expected to undergo significant transformation as a result of AI, with 26% of finance professionals identifying it as the area most likely to be impacted by AI over the next five years. This highlights a growing focus on utilizing AI to enhance the precision and adaptability of future-focused financial insights.
Fraud detection and prevention—a crucial advantage of AI as financial transactions become increasingly complex and dispersed— came in a close second (21%), demonstrating AI’s growing role in identifying patterns and flagging anomalies in real-time.
Meanwhile, 17% of respondents anticipate a significant transformation in accounts payable and receivable automation. Although many finance teams have already begun automating these processes, the next wave of innovation will likely focus on intelligent document processing, auto-reconciliation, and exception handling.
Finance functions most likely to be transformed by AI in the next five years
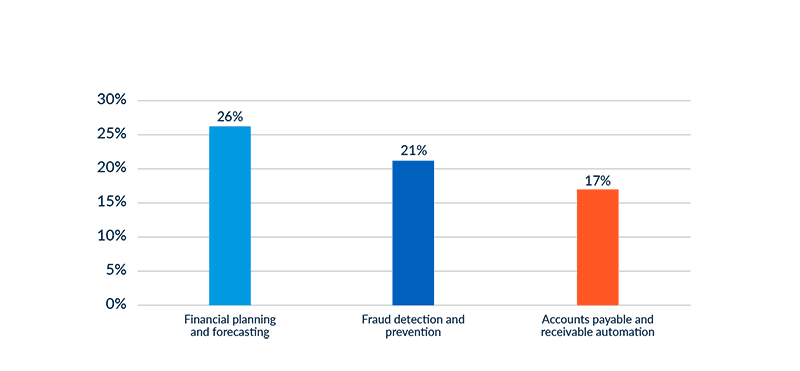
These findings reinforce a broader trend seen throughout the report: finance leaders are focusing first on AI use cases that offer immediate and measurable returns. But as adoption deepens, more transformative—and less expected—changes may be on the horizon.
AI’s greatest potential may lie in its ability to enable finance to respond more fluidly in dynamic business environments. Rather than reacting to events after the fact, finance teams will become equipped to operate in real-time and more accurately predict the future—adjusting forecasts, reallocating resources, and refining strategies as both internal operations and external market dynamics evolve.
This adaptability depends on AI’s ability to process data at a scale and speed that human teams alone can’t match. By continuously analyzing data, economic indicators, operational shifts, and market dynamics, AI allows finance to surface insights fast enough to guide action, not just explain what happened. Organizations that can recalibrate faster will be better equipped to navigate uncertainty and more prepared to turn disruption into opportunity.
Recommendations: Smarter AI for your finance teams
Start with a clear purpose
AI adoption should begin with intent, not implementation. Finance leaders must clearly articulate why AI is being introduced to their organization and what business outcomes it’s meant to support. Equally important is helping teams understand how AI will impact roles, processes, and priorities, making the “why” as practical as the “how.”
When teams understand AI’s value—whether through saved time, sharper insights, or higher-value contributions— they’re more likely to engage meaningfully, preventing misalignment and ensuring valuable AI outcomes.
Choose a platform that aligns with organizational priorities
Beyond end-to-end functionality, organizations should prioritize platforms with embedded AI features that accelerate adoption without requiring deep technical expertise and extensive customization.
However, current features are only part of the equation. Evaluating a vendor’s direction is equally essential to future-proofing your investment. A clearly defined product roadmap that demonstrates ongoing innovation and a commitment to intelligent automation signals a partner who can grow with your organization and support long-term business transformation.
Trust and transparency are important, especially for companies relying on third-party providers instead of building AI models in-house. Look for partners with robust data governance practices, regular security audits, and responsible AI policies that align with your organizational values. Efficiency and sustainability also matter— evaluate the computational footprint of the tools you use and choose vendors who share your long-term goals and business priorities.
Prioritize data quality before deployment
Data quality is one of the most significant barriers to successful AI integration. Finance teams often work with fragmented data sources, inconsistent field structures, and legacy systems that aren’t aligned with how AI models interpret information.
Before implementing any AI solution, organizations should assess where their data comes from, how it’s structured, and whether it aligns with the model’s logic. Addressing gaps may require data mapping, cleansing programs, or consolidating multiple sources into a standardized format. Centralizing these workflows improves visibility and control, enabling more advanced capabilities such as automated categorization, policy enforcement, and predictive cash flow analysis.
If foundational data is inaccurate or unstructured, AI will likely return flawed or misleading outputs. Treating data readiness as a prerequisite rather than an afterthought will significantly improve the effectiveness of your AI initiative.
Take a phased approach to implementation and oversight
Once the data foundation is in place, AI initiatives should begin with narrowly scoped, low-risk use cases. Focus on workflows where success can be clearly measured and outputs validated early. This phased rollout reduces risk while building momentum as teams learn and adapt.
The most effective AI programs follow a cycle of learning, validating, releasing, and expanding. In the early stages, teams should focus on observing how the model performs—learning from outputs, surfacing issues, and refining processes. From there, results can be validated through hands-on review and human oversight before being fully released. Once the system performs reliably, it can operate with less manual intervention and greater autonomy.
Only after these conditions are met should organizations consider expanding the use case or applying AI to more complex processes. This iterative approach helps mitigate risk, build trust, and ensure value is realized at every stage.
Balance automation with human judgment
AI delivers the most value when applied to high-volume, time-intensive processes that require precision and pattern recognition, such as forecasting, expense analysis, and spend optimization. Automation eliminates manual effort in these areas and uncovers insights that may otherwise be missed.
Still, automation isn’t a substitute for human oversight. Finance teams must be equipped not just to use AI but to engage with it thoughtfully, knowing when to rely on outputs, question them, and override automated decisions. That requires continuous investment in upskilling, with a focus on technical proficiency and adaptive thinking.
When human expertise remains central throughout the process, AI becomes an augmentation tool, not a replacement. It shifts finance roles toward more strategic, high-value work while automation handles the repetitive tasks. This partnership between people and technology ensures finance retains control, accuracy, and accountability throughout the process.
When you do all the manual work to analyze your spend, you typically find areas you can optimize. But when you leverage AI to make that entire process more efficient—from analyzing expenses across T&E, AP, and payments data— you’re saving time and unlocking insights that drive better decisions. That’s where you’ll often see an outsized return from your AI investment.
Adriana Carpenter
CFO
Emburse
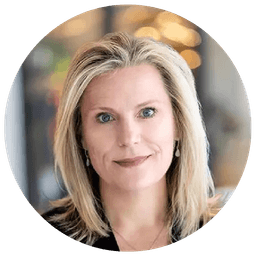